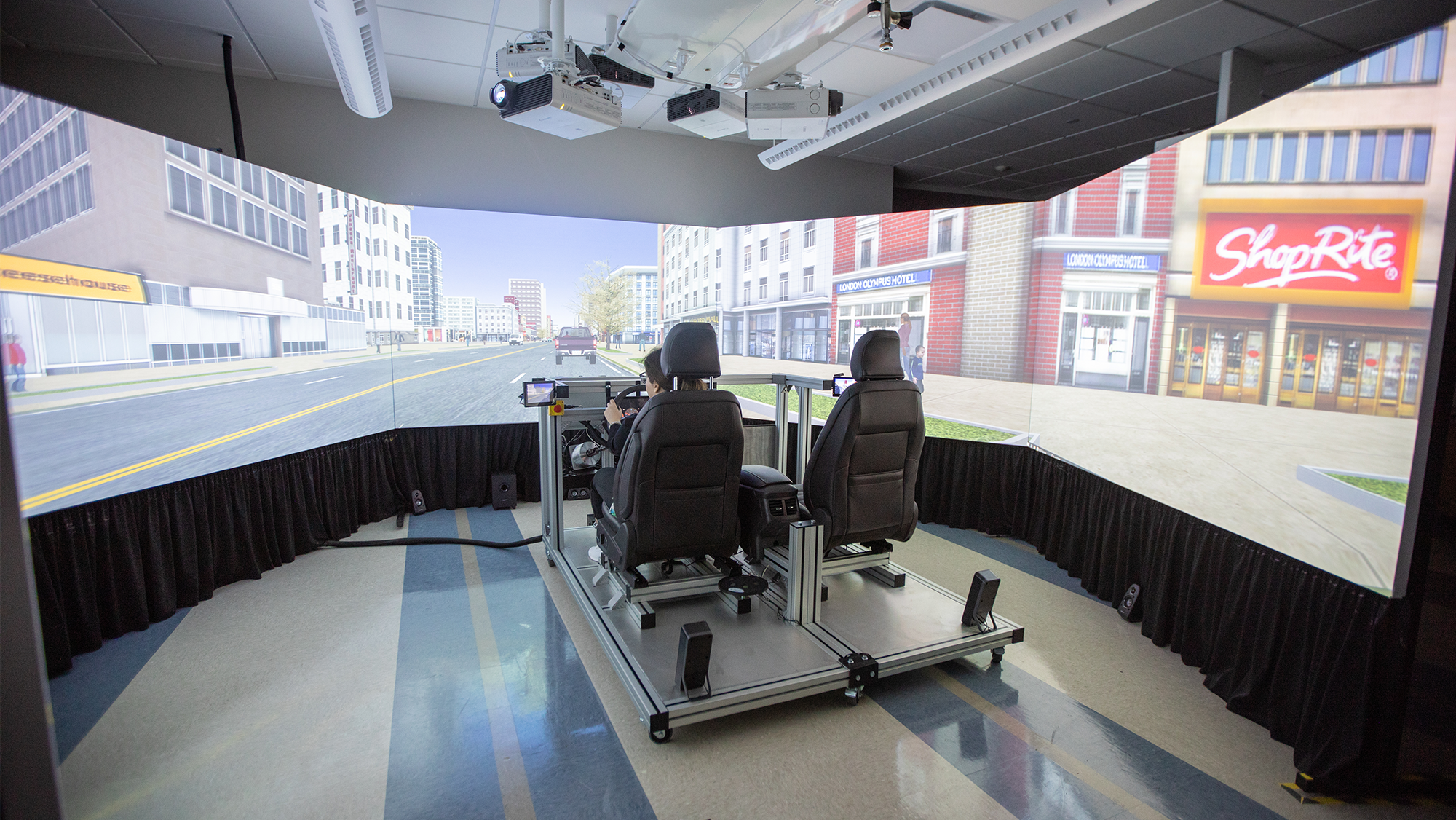
Motor vehicle crashes are one of the leading causes of death for law enforcement officers in the line of duty, accounting for almost 40% of fatal work injuries.
A principal reason this statistic is higher than the national average is that these crashes often include the use of in-vehicle technologies while driving.
“Motor vehicle crashes are a major cause of law enforcement officers’ injuries and deaths in the line of duty,” Zahabi said. “The main contributors to these crashes include officers’ use of in-vehicle technologies while driving, fatigue and lack of sufficient training in handling high-demand situations.”
Researchers at Texas A&M University are monitoring law enforcement officers’ driving workload to develop in-vehicle technologies and training solutions that will adapt to an officer’s workload to reduce the risk of crashes in law enforcement operations.
Dr. Maryam Zahabi, assistant professor in the Wm Michael Barnes ’64 Department of Industrial and Systems Engineering, received a 2021 National Science Foundation’s (NSF) Faculty Early Career Development (CAREER) Award for her research, “Adaptive Driver Assistance Systems and Personalized Training for Law Enforcement Officers.”
This project employs a combination of human performance modeling approaches, machine-learning algorithms, and behavioral and physiological measures in a hybrid algorithm to provide adaptations in real time.
The CAREER Award is the NSF’s most prestigious award that supports early-career faculty who have the potential to become role models in both research and education at their respective institutions.
The goal of Zahabi’s research is to provide law enforcement officers with real-time in-vehicle technology and driver-training adaptations to improve the driving training process and establish a strong education program model to train incoming law enforcement officers.
This research also paves the way for the next generation of students and scholars in human-computer interaction research.
The project will be conducted in three phases. During the first phase, the team will be administering ride-along observations with law enforcement officers to build human-performance models based on the data collected.
Phase 2 will use these models and algorithms to provide a real-time adaptation of in-vehicle technologies based on a driver’s cognitive state, which the team will evaluate using a driving simulation experiment.
In Phase 3, the team will create a flexible driving simulation-based training for law enforcement officers to improve the speed at which driving and interaction with in-vehicle technologies can be mastered. The team will then compare the effectiveness of this training with conventional, non-adaptive training approaches that are currently used in law enforcement agencies.
“We are in the first phase of this project and are developing the human-performance models for novice law enforcement officers using R software, which is a free, open-source and platform-independent software package,” Zahabi said. “We are planning to evaluate the performance of these models with the data that we will collect from the ride-alongs.”
This research aims to fill three gaps in particular: creating a holistic human-performance model of an individual’s cognitive state, expanding on the devices used to monitor a driver’s cognitive state and developing an adaptive driving simulation-based training system.
Human performance models have not provided a comprehensive evaluation of the human cognitive state, and applications for these models are still limited to normal driving conditions and are unable to model time-sensitive situations that many law enforcement officers face in their everyday jobs.
The research team will use a combination of cognitive models, machine-learning algorithms, and real-time physiological and behavioral data to provide a more comprehensive adaptive system.
“Previous examinations on adaptive training are generally a proof of concept without implementation in actual training settings, which might have been due to the limitations of off-the-shelf training media. We will fill this gap by developing an adaptive driving simulation-based training system,” Zahabi said. “This CAREER research seeks to fill this knowledge gap by establishing novice law enforcement officers’ performance models that can accurately represent their cognitive, perceptual and motor demands while driving.”
One-of-a-kind driving simulator
The Department of Industrial and Systems Engineering at Texas A&M University installed a new driving simulator to use in research pertaining to driving, autonomous vehicles and innovative research like Zahabi’s. It is a one-of-a-kind feature on campus that can be driven manually or autonomously, with a 270-degree field of vision. Due to the many different types of research that might require a driving simulator, interdisciplinary teamwork is almost inherent in any project that incorporates this technology.