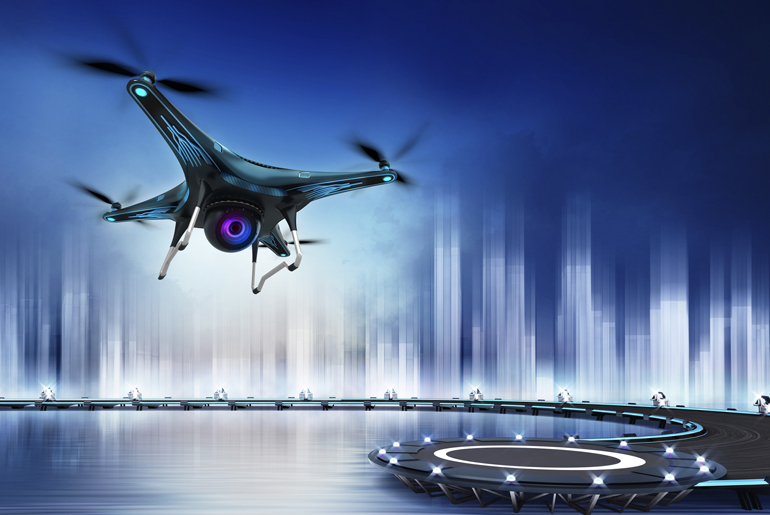
If you’ve ever used a smartphone or a fitness tracker, you’ve likely been using a device that’s a part of the internet of things — a group of interconnected devices that communicate via remote servers. But these central servers process huge amounts of data coming in from multiple devices that in turn cause processing delays, diminishing user experience.
To boost data processing speeds, Dr. Zhangyang (Atlas) Wang, assistant professor in the Department of Computer Science and Engineering, has been awarded two grants from the National Science Foundation (NSF) for developing highly efficient and energy-saving machine learning algorithms for use on local devices rather than on remote servers.
“We’d like to develop algorithms that make devices like your phone run faster, have low memory use, and be more energy efficient,” Wang says. “So, if you want to use your iPhone to take a photo with a facial recognition software, for example, energy efficient algorithms like the ones we develop are what ensure your battery doesn’t drain within five minutes.”
Wang’s first project, “Enabling Intelligent Cameras in Internet-of-Things via a Holistic Platform, Algorithm, and Hardware Co-design,” was awarded by the NSF Energy, Power, Control, and Networks program and is a collaboration with Yingyan Lin and Richard Baraniuk from Rice University. This project will improve machine learning algorithms for object recognition and other computer vision applications such as traffic monitoring and self-driving cars. Particularly, Wang’s team will optimize a type of machine learning algorithm called deep neural networks to process images.
For the second project, “Harmonizing Predictive Algorithms and Mixed-Signal/Precision Circuits via Computation-Data Access Exchange and Adaptive Dataflows,” Wang’s research team has been awarded a total of $1.38 million from the NSF Real-Time Machine Learning (RTML) program. With collaborators Lin and Baraniuk from Rice University, Boris Murmann from Stanford University and Yiran Chen from Duke University, Wang aims to enhance machine learning programs by training these algorithms in real time for a particular task, like object recognition. This approach will make the algorithms progressively better at doing the task over time.
One of the many applications of his research is drone-based object detection systems that can learn new environments without human supervision. “If we think of monitoring wide terrains for rescue operations, drones using our algorithms will be able to survey a large area more efficiently while continuously learning the layout of the new environment,” Wang says.
When their algorithms are ready for public use, Wang and his collaborators plan to post them on OpenStax, a nonprofit online education company created by Baraniuk, so as to lower the cost for people to access educational resources and encourage a global effort to address challenges in developing and improving software related to computer vision.
The NSF and Defense Advanced Research Projects Agency (DARPA) have teamed up to issue these grants through the RTML crosscutting program to explore high-performance, energy-efficient hardware and machine learning architectures that can learn from a continuous stream of new data in real time. The RTML program is a part of DARPA’s Electronics Resurgence Initiative, a five-year, $1.5 billion investment in the future of domestic, U.S. government and defense electronics systems. Out of over 100 submissions, Wang’s team was one of only six selected for large grants, further solidifying his group’s leading role in the machine learning field.